Dana Dawson, Ph.D
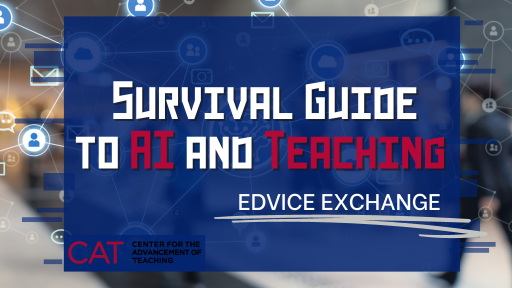
In previous posts in this series, we noted how generative AI can perpetuate biases and exacerbate the digital divide. Here, we will explore in more depth the potential for these tools to widen the institutional performance gaps that impact learning in higher education, but also the potential for generative AI to create a more equitable learning environment. We conclude with suggestions for what you can do to minimize possible negative impacts of generative AI for students in your courses.
Rapid improvements in the capabilities of generative AI have a tendency to provoke doom spiraling, and there are indeed some very real concerns we will have to grapple with in coming years. While generative AI at times produces helpful summaries of content or concepts, it is prone to error. Students with tenuous confidence in higher education or their capabilities of succeeding in their studies are less likely to deeply engage in their coursework (Biggs, 2011; Carver, 1998) and may rely excessively or uncritically on AI tools. Over-reliance on generative AI to reduce effort, and not as a mechanism for jumpstarting or supporting conceptual work robs students of opportunities to practice and develop the very creative, critical thinking and analysis skills that are likely to become increasingly valued as AI is more widely available. In addition, where we neglect to carefully vet content created by AI, we run the risk of repeating erroneous information or perpetuating disinformation. The prospect of bias and stereotypes impacting students’ experience in higher education arises not only from the content generative AI produces (Bender et al., 2021; Ferrara, 2023), but from the challenge of determining whether a student has appropriately used the tools. AI detectors cannot reliably differentiate human- from AI-generated content. Faculty must be aware that judgments of whether students relied excessively on AI may be influenced by assumptions that have more to do with factors such as race, gender or spoken language fluency than student performance. Finally, faculty who wish to encourage students to experiment with and integrate the use of AI tools must be aware that inequitable access to broadband internet connection and digital tools, along with varying levels of preparation to effectively use the tools, may differentially impact students. Variable access to broadband prior to, or during their postsecondary studies raises digital equity concerns. Some students will come to our classes well-equipped to engineer prompts and vet generated content while others will be encountering these technologies for the first time. That high quality AI applications are often behind paywalls compounds these issues.
On the other hand, some scholars and policy-makers have pointed to ways that these tools can be productively used to support student learning and success. AI tools such as ChatGPT can be used to fill in knowledge gaps related to a field of study or to being a college student more generally that are particularly salient for first-generation students or those whose previous educational experiences insufficiently addressed certain skills or topics. GPT3 responses to prompts such as “What are the best ways to study?” and “How do I succeed in college?” generate strategies that are useful and can be expanded upon with additional prompts. Warschauer et al. point out that for second language learners, the ability to quickly generate error-free email messages or to get feedback on one’s writing reduces the extra burden of studying disciplinary content in a second language. Students can prompt generative AI tools to explain concepts using relatable analogies and examples. For students with disabilities, generative AI can serve as an assistive technology, for example by improving ease of communication for those who must economize words, assisting with prioritizing tasks, helping practice social interactions or modeling types of communication.
RECOMMENDATIONS
1. Reduce the potential for bias to impact your assessment of unauthorized student use of generative AI tools by determining the following before the start of the coming semester:
- Which assessments have the most potential for unauthorized use?
- Is there an alternative mechanism for assessing student learning for those assessments most prone to unauthorized use?
- What are my guidelines for appropriate use of generative AI tools in this class?
- Can I reliably detect inappropriate use?
- Is my determination of inappropriate use subject to bias?
- What will my next steps be if I suspect inappropriate use?
If you’re not sure whether to allow use of generative AI tools, review our decision tree tool.
2. Clearly communicate your classroom policies on use of generative AI and talk with (not to) your students about those policies, ensuring they understand acceptable limits of use.
3. If you are encouraging the use of generative AI tools as learning tools, consider questions of access by:
- Assessing the extent to which your students know how to use and have access to the tools; and
- Showing students how to use the tools in ways that will benefit their education (for example, using follow-up prompts to focus initial queries). Temple University Libraries has created an AI Chatbots and Tools guide to help our students learn to judiciously use these tools.
4. Educate students on how generative AI tools may be biased, can perpetuate stereotypes and can be used to increase dissemination of mis- and dis-information.
- See the ideas in our previous blog post in this series and check out Temple Libraries’ “Fake News,” Misinformation & Disinformation library guide and How to SIFT Through Misinformation online tutorial.
5. Help students find their own voice and value a diversity of voices in writing and other content that has the potential to be generated by AI tools.
- Discuss the risks of homogenization of ideas and ways of expressing ideas that arise with the use of generative AI tools.
6. Consider a SoTL project.
- Cecilia Ka Yuk Chan points out that, “Regular assessments of AI’s impact on teaching practices and student performance will help identify areas for improvement and ensure that the technology is being used effectively and ethically.” Make use of the CAT’s umbrella IRB and other Scholarship of Teaching and Learning resources to support your projects.
In the next (and final) installment of our series, we’ll focus on how to talk to your students about generative AI. In the meantime, if you’d like to discuss AI or any other topic related to your teaching, please book an appointment for a one-on-one consultation with a member of the CAT staff.
Works Referenced
Bender, E. M., Gebru, T., McMillan-Major, A., & Shmitchell, S. (2021, March). On the dangers of stochastic parrots: Can language models be too big? In Proceedings of the 2021 ACM conference on fairness, accountability, and transparency (pp. 610-623).
Biggs, J. (2012). What the student does: Teaching for enhanced learning. Higher education research & development, 31(1), 39-55.
Carver, C. S., & Scheier, M. (1998). On the self-regulation of behavior. Cambridge, UK: Cambridge University Press.
Ferrara, E. (2023). Should chatgpt be biased? challenges and risks of bias in large language models. arXiv:2304.03738.
Dana Dawson serves as Associate Director of Teaching and Learning at Temple University’s Center for the Advancement of Teaching