Stephanie Laggini Fiore, Associate Vice Provost
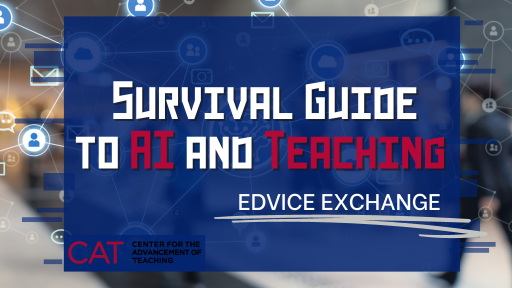
Even if you’ve done your due diligence in clarifying acceptable use of AI in your course, you may still suspect that students are using these tools in unauthorized ways. While unauthorized AI use is not considered plagiarism, it is still cheating and a violation of the university’s standards on academic honesty, as it both uses “sources beyond those authorized by the instructor in writing papers, preparing reports, solving problems, or carrying out other assignments” and engages “in any behavior specifically prohibited by a faculty member in the course syllabus, assignment, or class discussion.” The sticky question is, therefore, “How can I be sure that students have indeed inappropriately used these tools to complete their work?” We may be tempted to lean on detection methods as a solution, but is that the answer to this conundrum?
Can Humans Detect AI Work Unaided?
In playing with AI tools, you may have noticed some quirks in the output they provide (based on your prompts): they can be repetitive, go off on tangents unrelated to the topic at hand, or simply produce generic or illogical text. Generative AI can also “hallucinate” citations or quote text that simply doesn’t exist. These “AI tells” can sometimes tip us off to unauthorized AI use by our students. But how good are we at accurately identifying these tells? Our colleagues at the University of Pennsylvania conducted an investigation into human ability to detect AI text. They found that participants in their study were significantly better than random chance at detecting AI output but that there was large variability in ability among the participants. The good news is that their findings suggest that detection is a skill that can be developed with training over time (Dugan et al., 2023). At this point, however, few of us have had the targeted training referenced by the authors nor have we been able to dedicate the time necessary to improve. Barring glaring hallucinations or illogical content, most of us are simply not yet familiar enough with the features of AI text to be confident that our hunches are accurate. Try the test the researchers used; you may find, like me, that identifying AI text can be pretty darn challenging. And, of course, these tools will continue to evolve and improve, so our ability to detect non-human content may dwindle as generative AI advances.
Can AI Detectors Do the Job?
Don’t we all wish that AI Detectors (such as Turnitin, GPTZero, Copyleaks, or Sapling) were the answer to all of our generative AI concerns? Sadly, the simple and definitive answer to whether AI detectors can reliably detect AI-generated writing is “not at this time.” The reality is that these detector tools are flawed, delivering both false positives and negatives. In addition, unlike plagiarism detection tools, there is no way to verify that the detector’s conclusions are correct as the results do not link to source material in the same way. The CAT and the Student Success Center are conducting an investigation into error rates in a variety of AI detectors; early indications are concerning. In the meantime, others have pointed to the unreliability of the tools in both formal and informal investigations (here’s another), and in explanations of why these tools fail. Companies creating AI detectors themselves include disclaimers such as Turnitin’s statement that it “does not make a determination of misconduct…rather, we provide data for educators to make an informed decision.” They then go on to advise us to apply our “professional judgment” to these situations. That professional judgment, though, can itself be flawed.
Some faculty have been advised to run student work through multiple detectors, but the potential for (both positive and negative) bias may come into play as we make decisions about which detector to believe when they return different results (which, from our experience, they most likely will). My wonderful student couldn’t possibly have used AI so I believe the detector that says it’s human-written. OR I don’t doubt for a minute that this student cheated, so I believe the detector that says it is AI-written. Importantly, these detector tools can’t tell us if students have used AI in the ways we have outlined in our syllabi as permissible. Let’s say I am allowing students to use AI for idea generation or for writing an outline, but not for writing full drafts of papers. The detector cannot tell me whether students have used AI in permissible ways. Finally, there are already hacks out there with advice on how to beat the detectors; for example, videos that demonstrate how to run AI-generated content through a rephraser in order to fool AI detectors. All this adds up to inconsistent and unreliable results whereby catching those who have engaged in academically dishonest behavior is hit or miss and does not provide incontrovertible proof of misconduct. Most importantly, we have to consider the very real and potentially damaging effects of wrongfully accusing students of cheating when they have not.*
What’s a Harried Faculty Member To Do?
If detectors aren’t reliable and our own skills at detecting AI writing are not mature, what’s the answer? While we will all be adjusting to this new reality for a while, we can keep some fundamental principles in mind to nudge our students towards transparency and academic honesty, the first of which is to give up on a surveillance mentality as it simply won’t be effective (and you don’t want to police students anyway, right?). Instead, think developmentally and pedagogically by taking these steps:
1. Shift from a reactive to a proactive stance. Test your assessments in a generative AI tool to see how vulnerable they are to AI use. Then make some intentional decisions about whether to change assessments or create new ones. In the long run, of course, it is all about our assessments. We may have used these same types of assessments for decades, but they simply may not work in the way we want them to in the age of AI. Review blog posts #4, #5 and #6 to think about changes you may make to your assessments, or if you missed our Using P.I. to Manage A.I. series, see our suggestions there. Remember you can also make an appointment with a CAT developer to help you think this through.
2. Put a statement about AI in your syllabus clarifying acceptable use of AI! I can’t repeat this enough. Our colleagues at The Office of Student Conduct and Community Standards have expressed to us that it is essential to have clear guidelines clarifying what is and isn’t acceptable use of AI in our courses.
3. Engage your students in a discussion about generative AI and academic integrity, including why you have set the standards you have in your course. Remind them periodically about the ethics of generative AI use. (Look for an upcoming blog post for guidance on how to speak with your students about AI.)
4. Design courses that reduce the factors that induce students to cheat. James Lang, in his excellent book Cheating Lessons: Learning From Academic Dishonesty, reminds us that the literature on cheating points to an emphasis on performance, high stakes riding on the outcome, an extrinsic motivation for success, and a low expectation of success as factors that promote academic dishonesty. The good news is that we know also from the literature on learning that evidence-based teaching practices such as formative assessments, scaffolded assignments, ample opportunity for practice and feedback, development of a positive learning environment, and helping students to find relevance and value in what they are learning will both deter cheating by reducing these factors, and improve learning. Need help in reducing the temptation to cheat? Make an appointment with a CAT developer.
5. Plan thoughtfully for how you will manage situations where you suspect unauthorized use of generative AI, starting with a conversation with the student. (We’ll include advice on how to speak to students in the aforementioned future blog post.)
There is no doubt that generative AI is a disruptor in the educational space. Our response to that disruption matters for learning and for our relationship with students. Let’s work together thoughtfully towards a productive and forward-looking response. The answer is not detection—it is development
*Note: If I haven’t convinced you to avoid these flawed detectors in accusing students of cheating, I agree with Sarah Eaton that it is essential to transparently state in your syllabus that you will be using detectors. Do not resort to deceptive practices in an effort to “catch” students. In addition, never use detectors as the sole source of evidence as, of course, the results may not be reliable.
Stephanie Laggini Fiore serves as Associate Vice Provost at Temple University’s Center for the Advancement of Teaching.