By Sydney Grimm
As introduced by my friend and colleague, English PhD candidate SaraGrace Stefan in her overview of the Banned Books Project, we at the Representation Lab are using computational text analysis to examine a corpus of over 1,600 banned books identified by PEN America. But while computational analysis allows us to learn about what goes on between a book’s covers, my undergraduate teammates, Abigail Corcelli, Kriti Baru, and I wondered how the strategies of distant reading might be applied to books’ exteriors. As a Visual Studies and English double major and class of 2024, it was a new idea therefore to undertake a task we as lifelong readers and students have been repeatedly told not to do: judge books by their covers.
Over a span of four months, we recorded a detailed breakdown of the visual and textual information found on every book in our corpus. This research arose in part out of a suspicion that the people challenging books in libraries and schools didn’t always read the titles they flagged as inappropriate. In these cases, analyzing a book’s cover gives us valuable insight into how a title is received on first impression, and may point to trends among banned titles distinct from but related to what can be discovered through text-mining.
Categorizing Book Covers
The process of logging a book cover’s features is involved and complicated. We began by asking general questions about book cover design, finding that all visual elements could be categorized as either figures (human or animal), objects, landscapes, or as non-representational textual/design elements. This became the first step of our logging workflow and determined what further lines of inquiry to pursue. For example, for covers depicting a figure (spoiler alert: 85% of them do), we then logged the apparent race and gender of all figures pictured, as well as the nature of their interaction—romantic, platonic, or ambiguous.
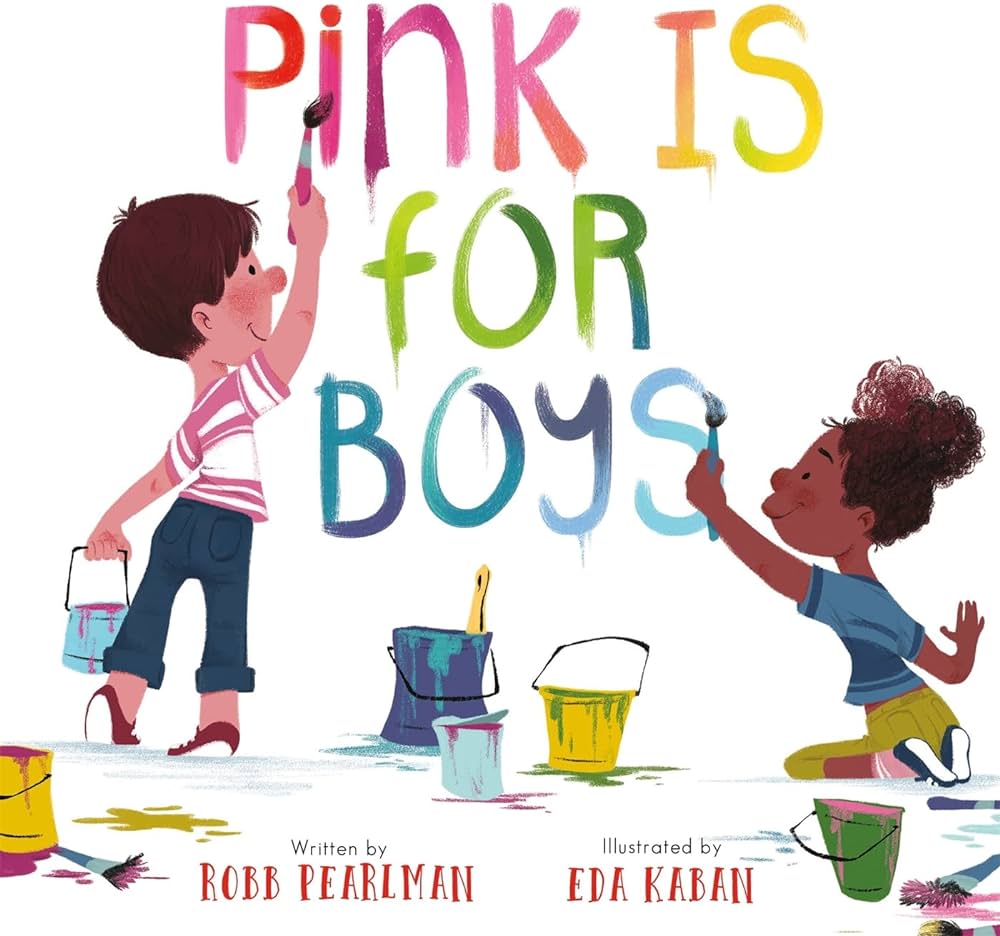
For all covers, we also made note of colors, some of which are clearly used with political implications—depictions of rainbows as a symbol of queer pride, or the association of the color pink with masculinity (see above).
The final step of the logging process involved identifying any words in titles or back-cover synopses that might raise challengers’ eyebrows, like those pertaining to sex, sexuality, or structural inequalities.
Data about each book was collected through a Google form, with results synched to save to a (very, very large) spreadsheet. The complete process is summarized in the graphic below.
While our methodology was mostly formalized by the time we sat down to log the first cover, issues arose intermittently that required us to rework and refine our original plans. Attempting to translate large amounts of qualitative data into something quantifiably measurable is always challenging, and this project was no exception.
The rest of this post will therefore provide a brief overview of the snags we encountered, how we accounted for them, and the potential impacts of these challenges on our results.
Self-Identification vs. Digital Categorization
One of our biggest concerns was categorizing figures based on their race and gender. We knew from the outset that the drop-down menus of our Google form—options of white, nonwhite, and ambiguous for race and male, female, and ambiguous for gender—were highly limited. For one, these categories are reliant on assumptions based on figures’ physical appearances. For another, they reinforce the idea that white/nonwhite and male/female exist as innate binaries and potentially disempower those who identify outside those binaries. However, we ultimately decided to limit our fields this way for three reasons:
1.) The introduction of too many variables would make it more difficult to deduce meaningful trends from the data.
2.) Greater specificity meant greater chance of misidentification. To gauge whether a figure appeared white/nonwhite or male/female was relatively simple in most cases, but to speculate about identity on a more granular level felt invasive and prone to greater error.
3.) Determining why a title might be banned often means analyzing it from the perspective of a book banner. We feel this movement operates in part out of a fear of minority representation and treats white, cisgender stories as the only stories worth telling. Our categories were therefore designed to reflect the reality that, in the eyes of challengers, all minority identities are viewed as homogeneously ‘deviant.’
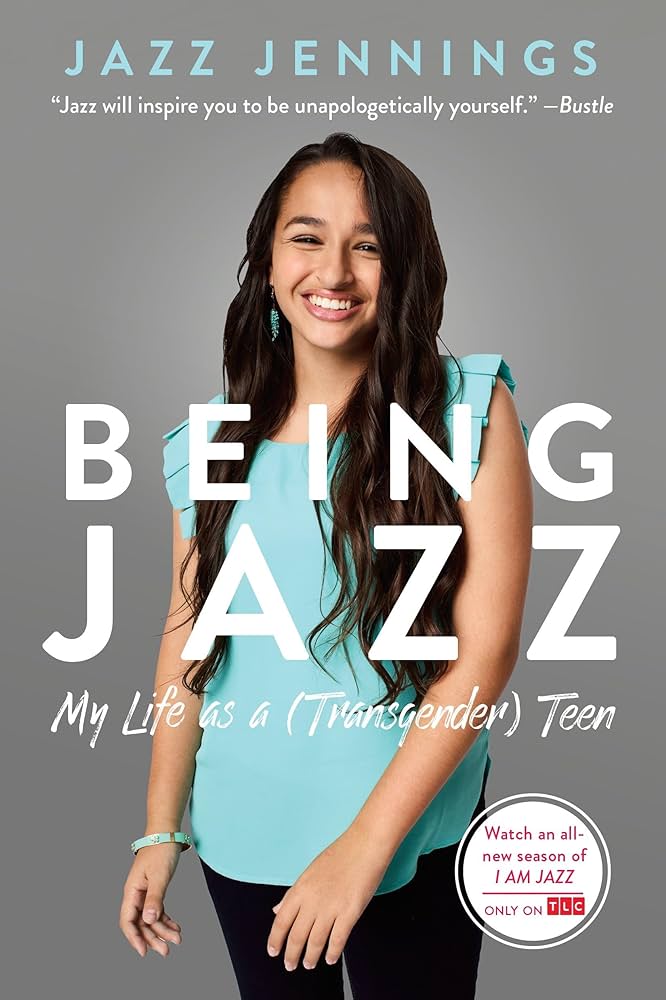
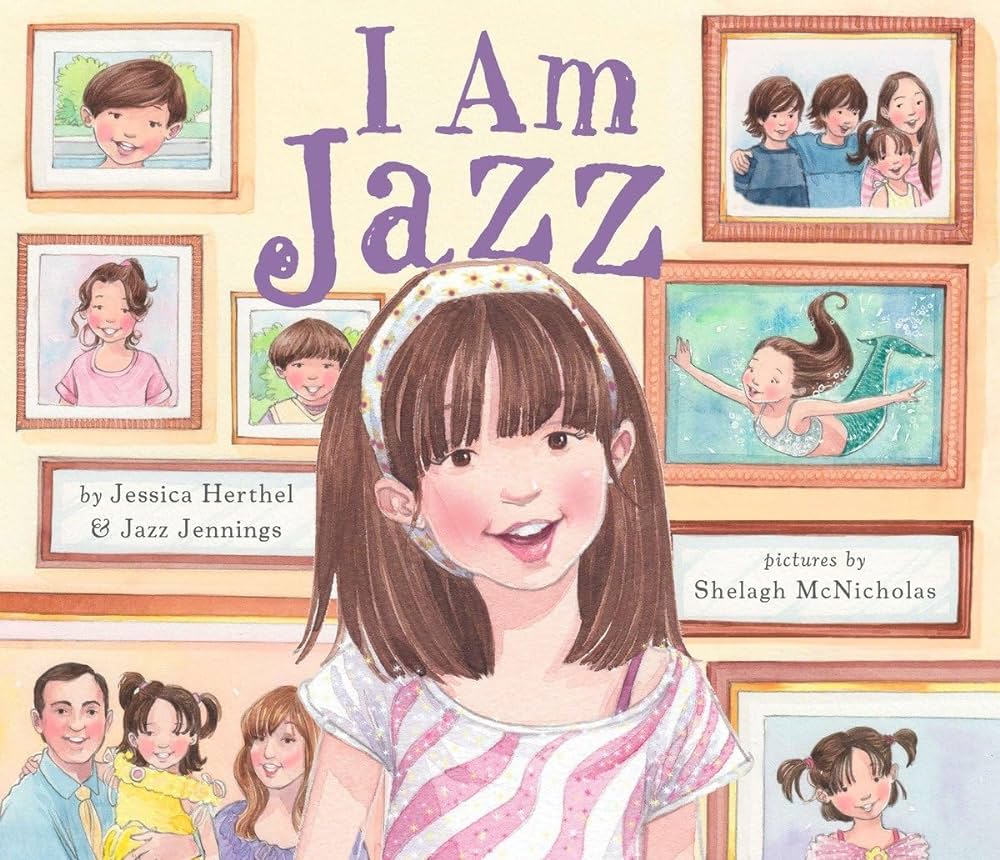
Limiting our fields in this way means that the information logged might differ from how a figure expressly self-identifies within the text. Information might even become contradictory if the same figure appears on multiple covers. As an example, above are two biographies of Jazz Jennings, one of which depicts Jennings’ with a considerably lighter skin tone than the other. Because our intent was to collect data free from context, assuming no prior knowledge of the text itself, we thought it reasonable to tag the illustrated Jennings as white and the photographed Jennings as nonwhite.
Denotative vs. Connotative Meaning
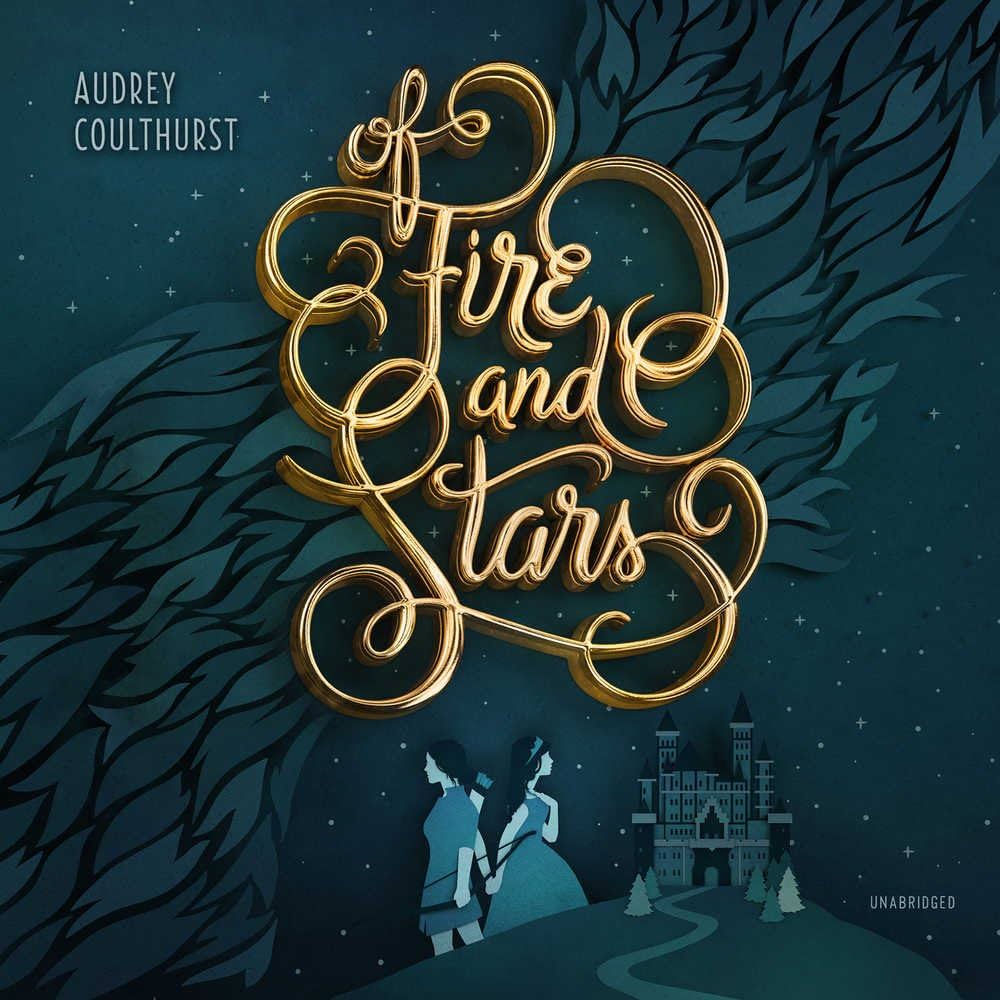
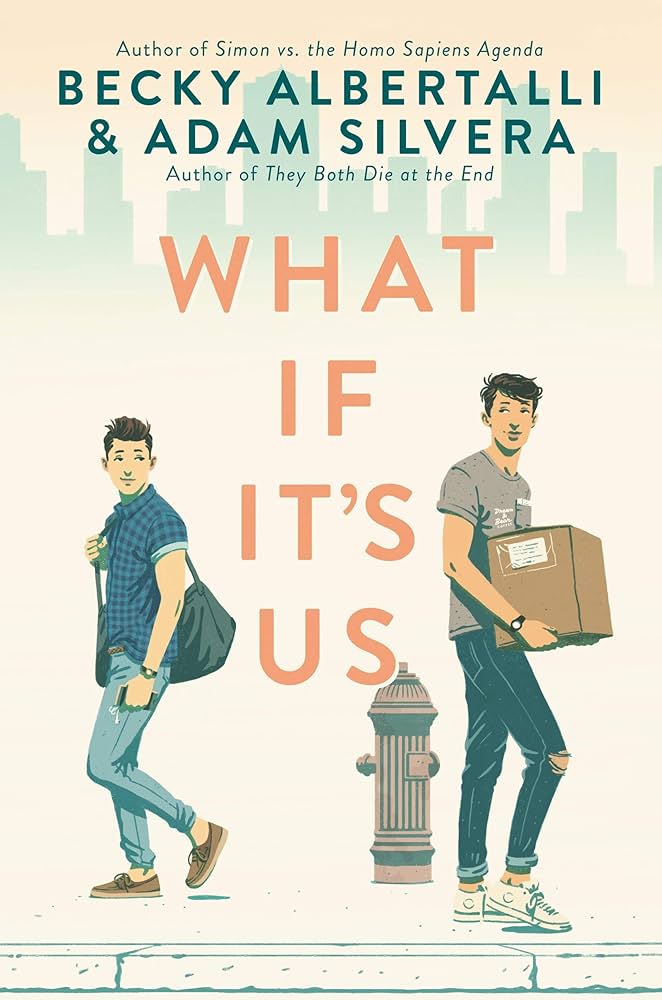
Another point of contention related to handling visual and written subtext. How, for example, do we determine whether the relationship between two figures is platonic or romantic? Above are two examples where this ambiguity proved a problem, sparking debate about whether hand holding and eye contact connoted romantic attraction.
In both cases, we decided to err on the side of skepticism and tagged the interaction romantic, believing that, to a challenger, even the potentiality of queer romance might prompt enough discomfort to warrant a ban.
The problem of subtext also arose when trying to mine keywords from the synopses on back covers. Keyword tagging only accounts for words that appear explicitly in print, but sometimes the kinds of “objectionable” content that led to a book’s banning are only alluded to obliquely, especially when it comes to historically taboo subjects like queerness.
Take for example this excerpt from the cover synopsis of Brent Hartinger’s 2004 YA novel Geography Club:
“Russel is still going on dates with girls. Kevin would do anything to prevent his teammates on the baseball team from finding out. Min and Terese tell everyone they’re just really good friends. But after a while, the truth’s too hard to hide.”
Queerness is clearly a theme of the novel, but nowhere is it overtly mentioned in a way that could be tagged as a keyword. This led to the creation of a separate notes field where we put any important information not otherwise accounted for.
Conclusions and Next Steps
While our dataset’s notes column serves as a successful workaround to account for certain forms of subtext, other issues, like the inherent problem of race and gender tagging, are inescapable. When developing a dataset through this methodology, these issues should always be considered critically, especially when trying to draw conclusions from the results.
However, it’s important to remember that the goal of this research project was less to document figures as they truly are and more to document the way they’ll be perceived by the public. In a way, our difficulty in categorizing a set of varied, ambiguous bodies speaks to the diversity of our corpus, and of the growing demand for minority protagonists in adult and especially YA literature. While they might be cumbersome to account for using our research methods, bodies that defy neat categorization represent those most under threat from book banners, and are therefore most needing of our advocacy.
Through the data collected, we’re able to visualize trends in our corpus, including the most common keywords found in books’ synopses, the percentage of covers that depict a figure, and the racial and gender breakdown of those figures. For example, over 60% of figures who appear alone on covers are female.
For more about the results of the Covers Project as well as what’s next for the Representation Lab, visit Abigail Corcelli’s blog post “Pulling Back the Curtain on Censorship: Part 3 of The Banned Books Project.”