By R.J. McGhee
Introduction
Have you ever wondered how music reviewers write about vocalists in their reviews? How do music reviewers depict the way vocalists sing? Which adjectives and verbs help create a depiction of vocal styles?
As a student in a master’s program in Information Science and Technology and a musician, my goal for the Cultural Analytics Certificate Practicum project is to use my background knowledge to explore how reviewers of music albums describe sound, specifically the description of the musical properties of vocal sounds. I selected a dataset of music reviews from the prominent music journalism platform, Pitchfork, for this analysis.
To analyze the words used to describe vocal sounds in Pitchfork music reviews, I employ natural language processing techniques, utilizing Python libraries like pandas
, and SpaCy
to tally a list of Tagg’s “Sound Descriptor Words” within the Pitchfork Reviews. To see my full code, follow this link.
Background:
In his book, “Music Meanings,” Musicologist Phillip Tagg investigates the ways we discuss music and its significance to the world (Tagg, 2013). Tagg identifies the phenomenon of individuals talking about vocals using the “Aesthetic Perspective.” Tagg defines the “Aesthetic Perspective” in terms of how vocal sounds are perceived, interpreted, and reacted to by listeners (Tagg, 2013).
Tagg created a list of ‘Directly Sound Descriptive Adjectives and Verbs’ to catalog the language commonly used when discussing the qualities of vocal sound. See his list below:
Sound Descriptor Word List:
hum, high-pitched, whiny, squeaky, booming, low-pitched, deep, full-throated, gruff, breathy, husky, guttural, distinct, harsh, indistinct, muffled, plaintive, rasping, roaring, shrill, stammering, loud, declamatory, soft, quiet, monotone, lispy, bird-like, hoarse, throaty, babble, bark, bawl, belch, bellow, bleat, blubber, boom, buzz, cackle, caterwaul, chant, chatter, chuckle, chirp, cluck, complain, cough, croak, croon, cry, declaim, denounce, drone, exclaim, gargle, gasp, giggle, growl, grumble, gurgle, hiccup, hiss, hoot, howl, lament, laugh, lilt, moan, mumble, mutter, praise, preach, proclaim, pronounce, quack, quip, rant, recite, roar, scream, screech, shout, shriek, sigh, snap [at], snarl, snigger, snore, snort, sob, spit, splutter, squawk, squeak, stammer, stutter, twitter, ululate, wail, warble, weep, wheeze, whimper, whine, whinge, whisper, whistle, whoop, yammer, yap, yawn, yell, yelp, yow
By examining the frequency of these Sound Descriptors and their association with genre categorization in Pitchfork reviews, I hope to find insights into whether and how reviewers are using sound descriptors in their writing. This involves tallying the occurrences of words from Phillip Tagg’s list in sentences discussing vocal sounds.
The Dataset
For my dataset, I downloaded a set of 24,169 Pitchfork reviews spanning from January 5, 1999, to December 12, 2021 that Nolan Conaway web-scraped from the Pitchfork website.
I chose this dataset because of its accessibility and its cultural importance to music journalism. Pitchfork is a cornerstone of the online music review culture. Pitchfork started in 1996 as a small music review website and has now grown to be one of the most well-known music review websites internationally (“The History of Pitchfork’s Reviews Section in 38 Reviews,” 2021).
The dataset can be downloaded for free as a SQLite3 file. The tables contain columns which include the name of the artists, the title of the album, the score, the author of the review, the primary genres, the release year of the album, the publication year, and the review itself.
Text Cleaning Process
My first step in this research project was to consolidate these tables into one functional table, which I can further filter to answer my research questions. My main avenue of organizing this data was to use a library in Python called pandas
. I first converted each table into pandas dataframes using SQL calls, which then I was able to connect each table together using their respective table keys. I then created a new pandas
dataframe by genre. The genres are assigned to every article in the dataset.
Pitchfork Genres
Experimental | Rock | Electronic | Folk/Country | Rap | Pop/R&B | Jazz | Global | Metal |
I also needed to prepare the data for analysis, so I applied SpaCy’s lemmatization feature to each sentence, facilitating the extraction of root forms of words. This step ensures the identification of terms from the sound descriptor and vocal term list, accommodating for the variations in word forms due to prefixes or suffixes (“Linguistic Features · SpaCy Usage Documentation,” n.d.).
Text Mining Process
To begin mining these texts, I needed to verify that I counted only instances where the sound descriptive words described vocal sounds. I created a basic list of words that would normally describe vocals and appear in reviews discussing vocals. See the list below:
- Vocal
- Vocalist
- Voice
- Lyric
- Speak
- Sing
- Sings
- Sung
- Sang
- Falsetto
- Rap
I segmented the reviews into sentences using the NLTK library and checked each sentence for the predefined nouns associated with vocals. If found, I added a boolean value to the dataframe.
Result
Once my data was cleaned and filtered, I searched through these reviews and counted the number of times the list of Phillip Tagg words occurred by genre. My code counts the number of times these words occur in each genre. I averaged the result using the total number of words in each genre to create a frequency ratio. See some examples of my results below:
Top 5 Vocal Sound Descriptors for Rock Genre
Word | Frequency | Frequency ratio (%) |
---|---|---|
Deep | 303 | .0092 |
Soft | 271 | .0082 |
Whisper | 210 | .0064 |
Scream | 204 | .0062 |
Drone | 189 | .0057 |
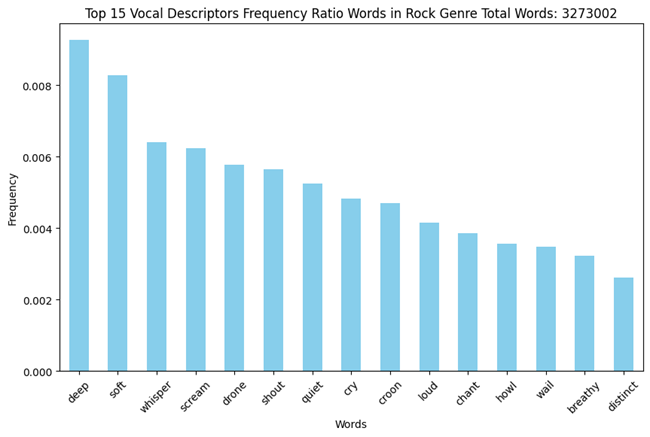
Top 5 Vocal Sound Descriptors for Folk/Country Genre
Word | Frequency | Frequency ratio (%) |
---|---|---|
Deep | 50 | .0162 |
Quiet | 43 | .0139 |
Soft | 43 | .0139 |
Whisper | 35 | .0113 |
Drone | 25 | .0081 |
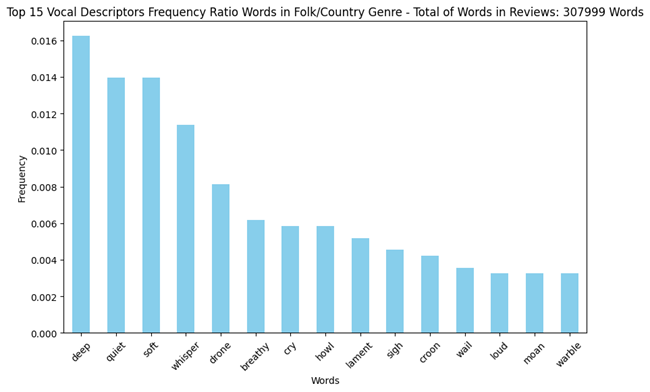
Top 5 Vocal Sound Descriptors for Rap Genre
Word | Frequency | Frequency ratio (%) |
---|---|---|
Deep | 132 | .0144 |
Spit | 90 | .0098 |
Boom | 82 | .0089 |
Shout | 58 | .0063 |
Soft | 52 | .0056 |
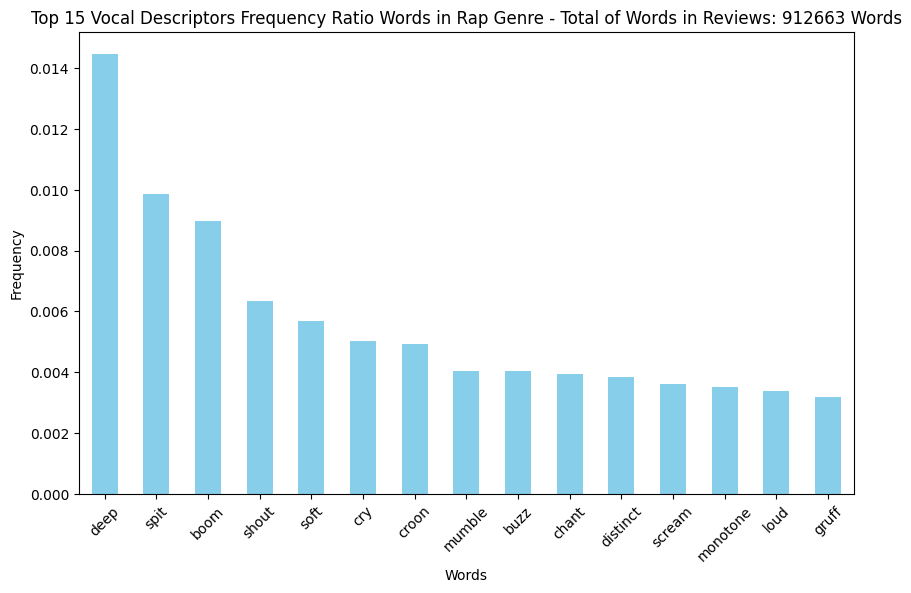
Top 5 Vocal Sound Descriptors for Jazz Genre
Word | Frequency | Frequency ratio (%) |
---|---|---|
Deep | 10 | .0076 |
Soft | 8 | .0061 |
Whisper | 8 | .0061 |
Scream | 6 | .0046 |
Drone | 5 | .0038 |
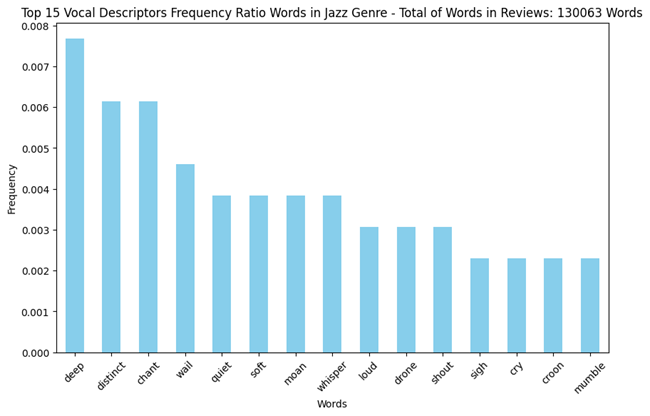
Top 5 Vocal Sound Descriptors for Global Genre
Word | Frequency | Frequency ratio (%) |
---|---|---|
Soft | 11 | .0186 |
Deep | 10 | .0169 |
Chant | 5 | .0084 |
Wail | 5 | .0084 |
Plaintive | 4 | .0084 |
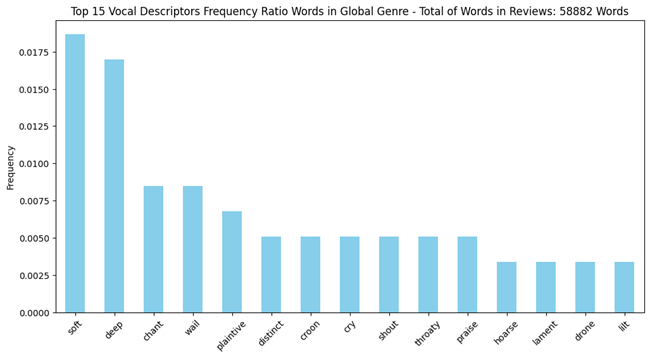
Top 5 Vocal Sound Descriptors for Metal Genre
Word | Frequency | Frequency ratio (%) |
---|---|---|
Scream | 42 | .0174 |
Growl | 36 | .0149 |
Howl | 25 | .0103 |
Shout | 25 | .0103 |
Deep | 25 | .0103 |
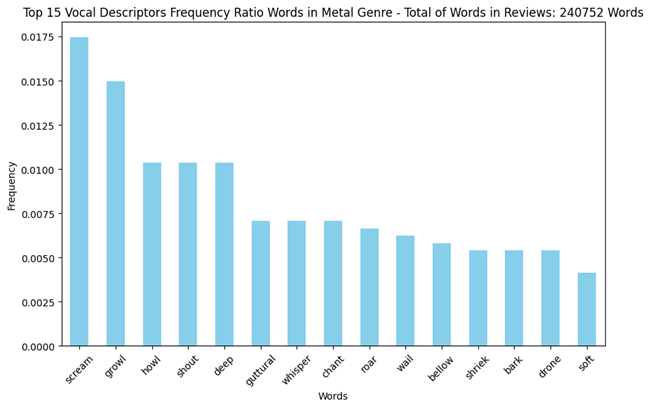
To see all of the results, please follow this link to access my Github page.
The term ‘deep’ appears as one of the top words in every single genre. This may reflect that the reviewers are referring to singers who use a ‘lower,’ ‘deeper’ voice. This may be due to the fact that Pitchfork is referring to a ‘deep’ voice, which is likely a musical expression that singers often use which stands out to reviewers.
After reviewing these top vocal sound descriptor words, the sound descriptors that come up the most tend to be descriptors that are often associated with the genres. For example, in folk, there are mentions of soft, whisper, and quiet, versus metal which contains scream, growl, howl, etc. I find this interesting, as the jazz and global genres contain words with vocals that tend to be soft and deep.
Conclusions and Next Steps
After analyzing these results, we can conclude that the list of Directly Sound Descriptive Adjectives and Verbs can be an effective way to identify terms that relate to vocal inflections and potentially build this list. We should continue to understand Phillip Tagg’s philosophy and aim to seek insights into the diverse ways in which vocal sound is commonly perceived and described in everyday contexts.
The next steps involve continuing to expand our list of words mentioned earlier. In Philip Tagg’s book, he mentions that this list is by no means comprehensive in describing every single word aesthetically for vocals. I am interested in further utilizing SpaCy’s part-of-speech tagging to identify other words used in the reviews and finding more words I could add to this list.
References
Linguistic Features · spaCy Usage Documentation. (n.d.). Retrieved from Linguistic Features website: https://spacy.io/usage/linguistic-features
Tagg, P. (2013a). Music’s meanings : a modern musicology for non-musos (p. 346). New York: The Mass Media Music Scholars’ Press.
Tagg, P. (2013b). Music’s meanings : a modern musicology for non-musos (p. 353). New York: The Mass Media Music Scholars’ Press.
The History of Pitchfork’s Reviews Section in 38 Reviews. (2021, May 25). Pitchfork. Retrieved from https://pitchfork.com/features/lists-and-guides/the-history-of-the-pitchfork-reviews-section-in-38-important-reviews/