Education researchers have noted the durability and persistence of socioeconomic inequalities in educational outcomes, a situation that has alternatively been called “Maximally Maintained Inequality” or “Effectively Maintained Inequality“.
Four years ago, my collaborator Jennifer C. Lee at Indiana University and myself were wrapping up an article on college enrollments and she brought to my attention a National Center for Education Statistics (NCES) data series showing that income inequalities in college enrollment among recent high school grads had dramatically reduced starting in 2014 where low-income young adults’ chances of enrolling in college shot up, completely eliminating the gap between themselves and middle-income young adults.
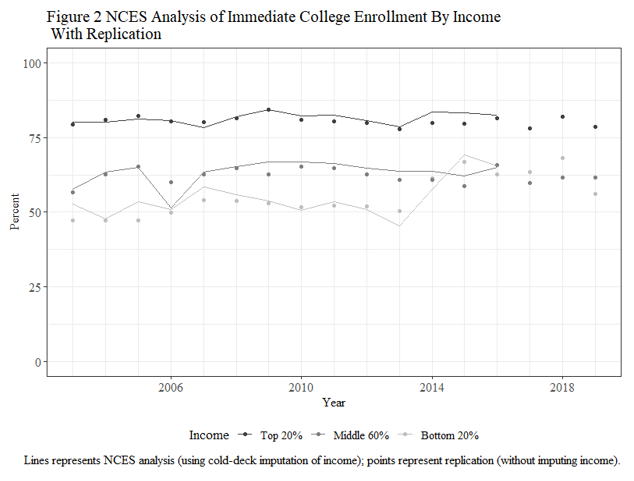
This was surprising to us, as it goes against the general thrust of MMI/EMI. Along with Genesis Arteta at Temple University, we investigated this finding in more detail. I am pleased to report that the fruits of this are forthcoming in Socius: Sociological Research for a Dynamic World (the article is not up yet, but a preprint is available on SocArXiv; and we are presenting this study on Sunday, August 7 2022 at 2pm in the LA Convention Center, room 153C at the annual meeting of the American Sociological Association).
There are problems with the data used to generate this data series, the Current Population Survey, and we are not the first to talk about this (indeed, NCES has discontinued the data series due to this problem). Namely, a good chunk (around 15 percent) of recent high school graduates are independent adults who are never observed living with their parents, and so we have no information on the incomes of their families of origin. We sought to get around this issue in two ways. First, we imputed the incomes of independent adults using other young adult high school graduates who were observed living with their parents, but subsequently left their parental household during the CPS data collection period. Second, we used an additional data source, the Panel Study of Income Dynamics – Transition Into Adulthood supplement (PSID-TA) which tracks individuals over their entire life course and does not run into the same issue of independent adults that CPS does.
Interrogating the NCES data series is worthwhile (it has informed policymakers), but we sought to make additional contributions to knowledge. First, we extend the data series to 2020, the first cohort of high school graduates navigating the decision to go to college or not in the COVID era, and we know little about how the pandemic has differentially influenced educational transitions by social class. Second, we examine not just college enrollment but also (using just the PSID-TA) degree attainment for the cohorts coming of age before and during the Great Recession.
We find that in both the corrected-CPS results and in the PSID-TA the low-middle income gap remains strong and statistically significant for the cohorts graduating from high school between 2014-2018. The gap may have weakened (we are not sure), but the original NCES analysis is definitely overstating the case in saying it completely disappeared. Income gaps in college enrollment also remained strong for the 2020 cohort, although curiously we do find that higher-income students were less likely to enroll in college which may have reduced the high-middle gap.
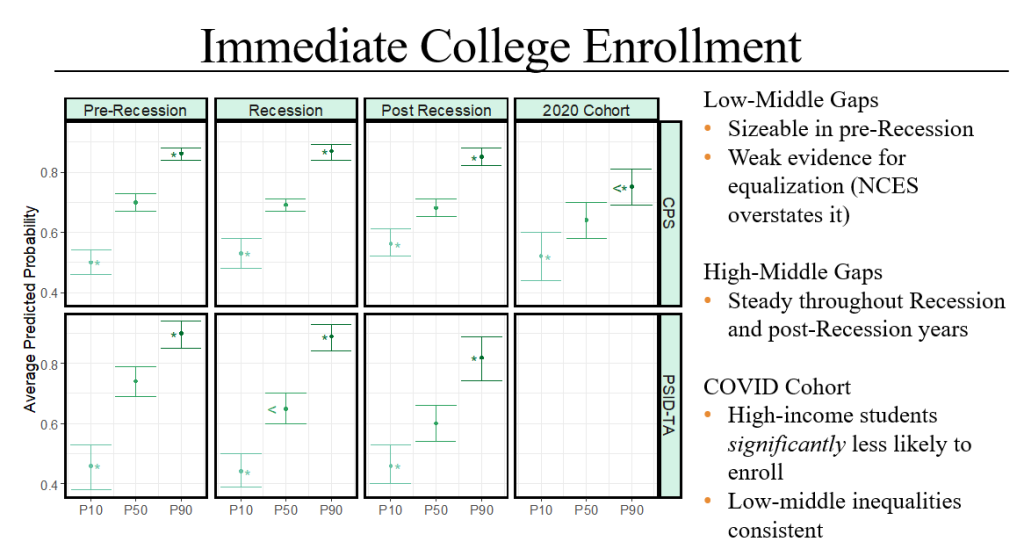
We also find that income gaps in getting a college degree (any degree, as well as a BA degree specifically) remained robust. If anything, it looks like the low-middle gap may have increased among college entrants for cohorts graduating from high school during the Great Recession years (as opposed to those graduating in the pre-Recession years).
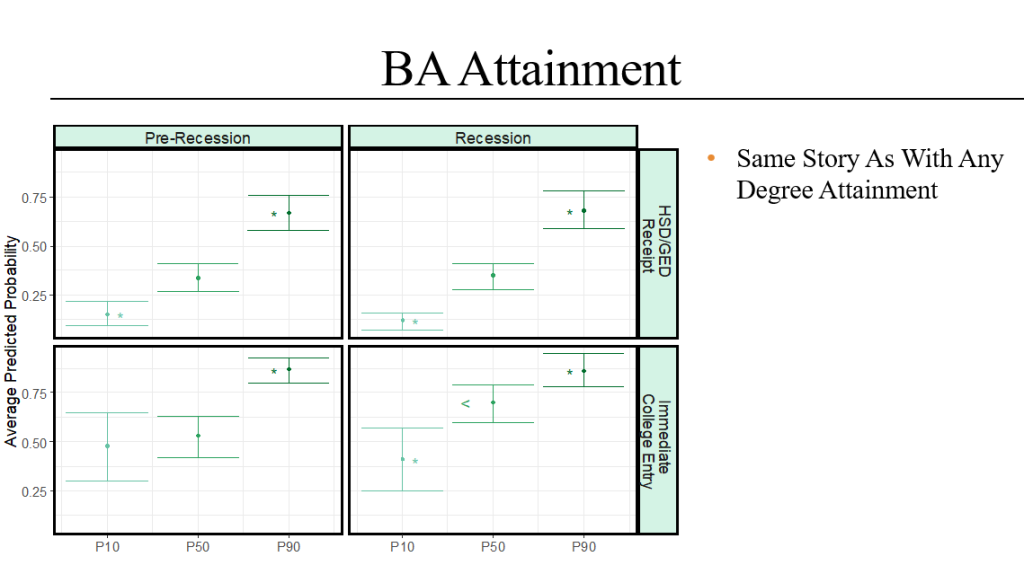
In short, we uphold the essential gist of MMI and EMI: class inequalities in college-going (as well as getting college-degrees) persist. There is some evidence that inequalities in enrollment weakened in the post-Recession and COVID eras, but it is weak. We see no evidence that inequalities in degree attainment have weakened for cohorts coming of age during the Recession.