Our new paper “Multi-ensemble Markov Models to estimate affinities and rates of ligand binding is now published in the Journal of Chemical Physics. Congrats to Yunhui Ge for getting this across the finish line!
This work examines a toy receptor made from an icosahedron of 11 Lennard-Jones particles. The ligand is a LJ particle, and all is solvated in explicit water. This system has number of encounter-complex states and a slow(ish) residence time of 30.3 ns.
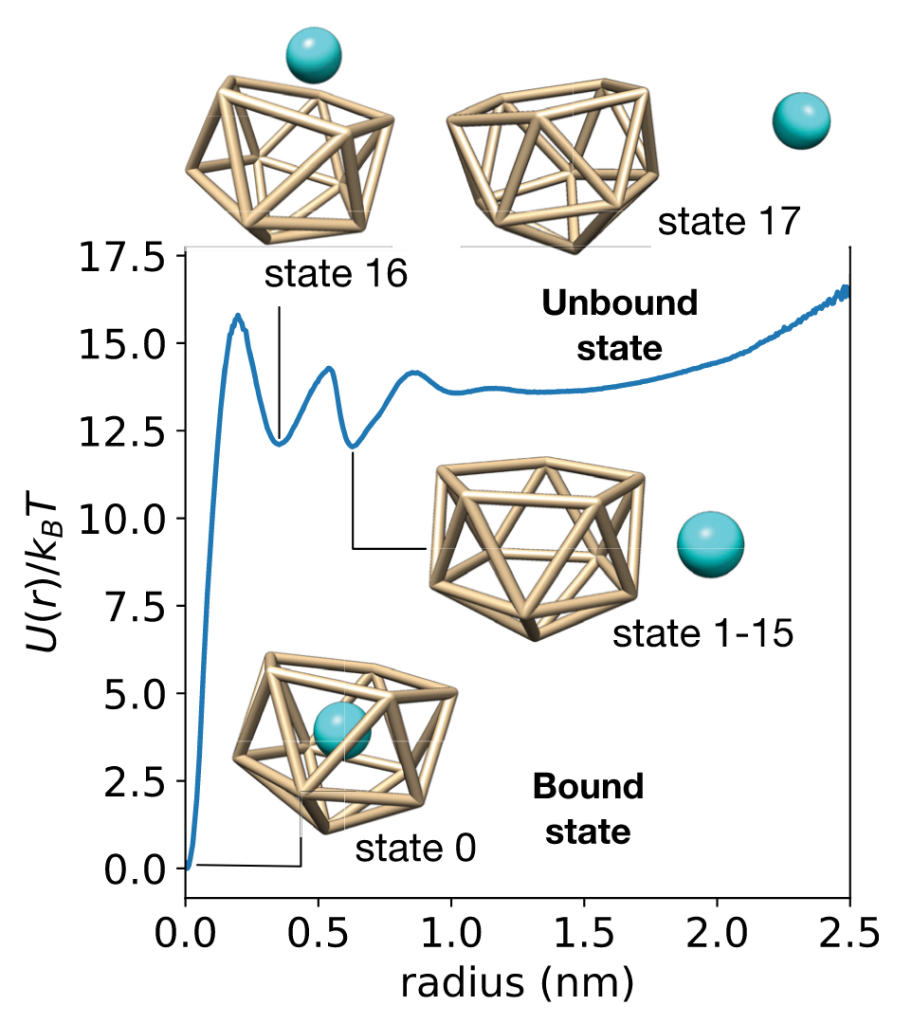
While conventional MSMs accurately estimate binding rates from swarms of short trajectories, they estimate affinities poorly. Why? Because MSM estimators typically enforce detailed balance, assuming the data is sampled at equilibrium.
So even with lots of parallel simulation, it still takes a long time for bound and unbound populations to equilibrate, and get a good model. (Our related work explored this problem with adaptive seeding simulations.)
Instead, what if we could quickly collect many binding/unbinding transitions in a biased ensemble, and use this information to infer populations and rates in the unbiased ensemble. This is a job for multiensemble Markov models, or MEMMs!
To accelerate transitions, we scaled the ligand nonbonded interactions, as is typical in FEP calculations for drug discovery. We tried two different MEMM estimators: (1) TRAM, from the Frank Noé group in Berlin, and (2) our Maximum-Caliber approach.
We find that both work pretty well (!), but TRAM works slightly better.
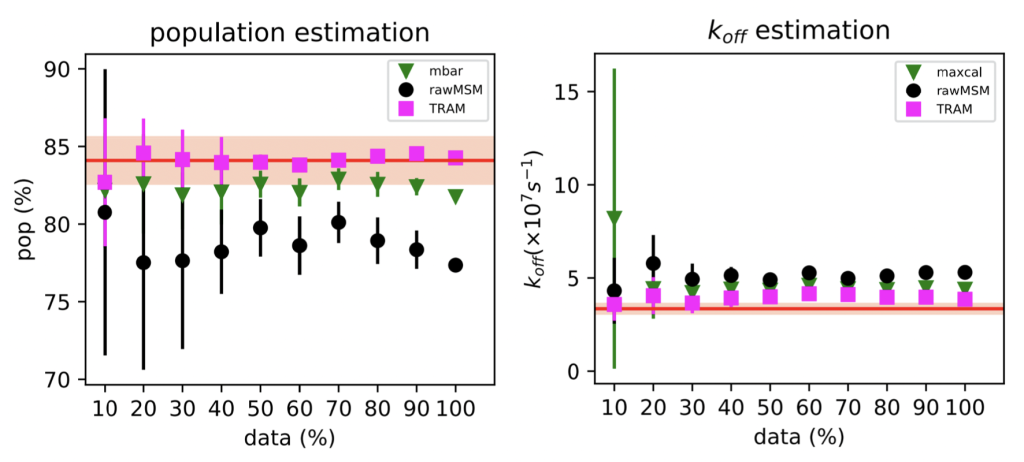
These results have encouraged us to explore how this method works in more realistic ligand binding systems, as a potential tool for MD-based virtual screening. Expect more from our lab on this soon
You can read more about this work here:
Estimation of binding rates and affinities from multiensemble Markov models and ligand decoupling. Ge, Yunhui, and Vincent A. Voelz. The Journal of Chemical Physics 156, no. 13 (April 7, 2022): 134115. https://doi.org/10.1063/5.0088024
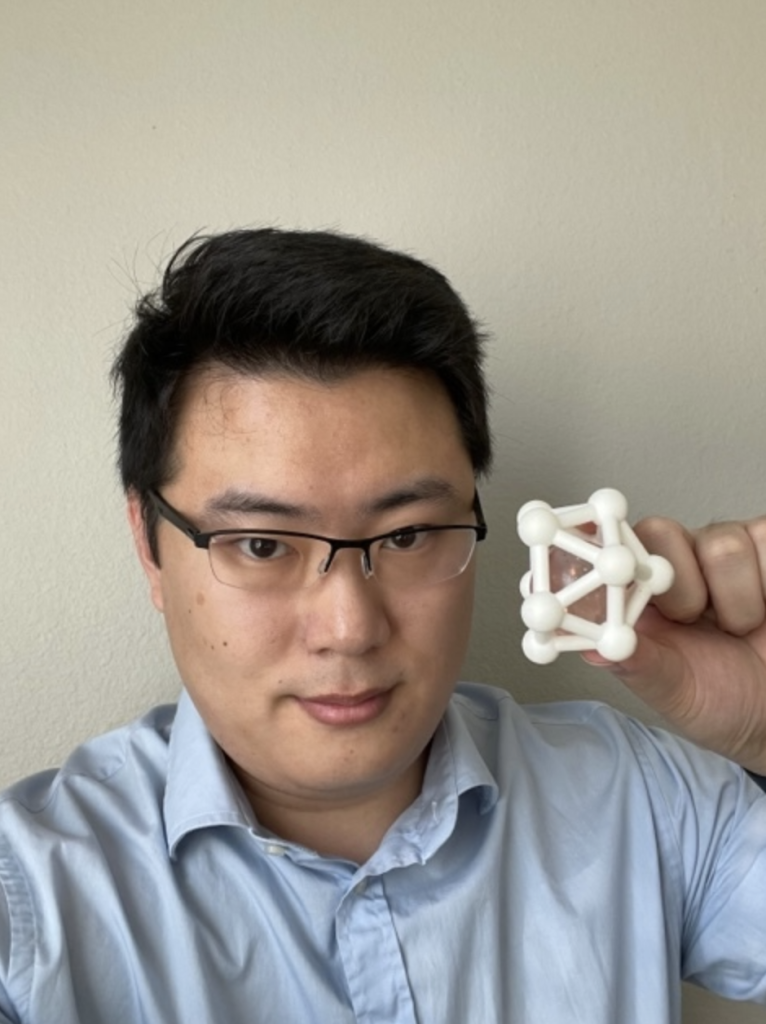
Yunhui Ge, holding a model of the toy binding pocket.