This paper presents a reduced-order modeling technique for energy storage systems (ESS) such as Lithium-ion batteries (LIBs). Data-driven models (DDMs) accurately represent the system dynamics without requiring the in situ measurements and proprietary information needed by physics-based models. However, the DDM sometimes result in poor performance in unseen scenarios as they tend to overfit the available data. Here, we present a novel data-driven modeling technique to discover the governing equations of individual cells using only the excitation inputs and measured outputs. Instead of adding generic terms to discover the model, we seek physics-informed reduced order nonlinear models. Our technique is based on Sparse Identification of Nonlinear Dynamics with Control (SINDYc) and was solved using Sequentially Thresholded Ridge regression (STRidge) optimization. The method accounts for the noisy data using Kalman filters, which update the terms for an enhanced state of charge (SOC) estimation. We propose using two scenarios as training and validation sets to tune the hyperparameters (threshold and regularization parameters) and a third scenario to validate the model (test set). The data for the system identification was generated using a high-fidelity model of a Li-ion cell. The model was trained on uniformly distributed electrical current signals with maximum amplitudes of 2C charge and 4C discharge rates. We used the US-highway profile (US06) as the validation set. The generalizability of the model was assessed with Urban Dynamometer Driving Schedule (UDDS) data where the identified model achieved the normalized root mean square error (NRMSE) of $8.3\times10^{-5}$ for SOC and Voltage predictions.
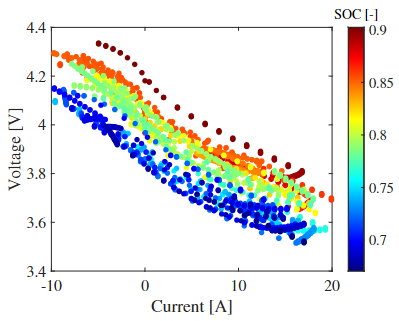
O. Ahmadzadeh, Y. Wang, and D. Soudbakhsh, “Sparse modeling of energy storage systems in presence of noise,” IFAC-PapersOnLine 56.2 (2023): 3764-3769
doi: https://doi.org/10.1016/j.ifacol.2023.10.1546