Accurate modeling of Lithium-ion batteries (LiBs) allows for more efficient utilization of their potential without compromising their safety or useful life. Accurate physics-based models require in-situ measurements and proprietary information unavailable for each cell. Data driven models offer a solution to identify governing equations of individual cells using only the excitation inputs and measured outputs. However, the main drawback of such models is their performance in unseen scenarios, as they tend to overfit the training data and perform poorly in other scenarios. We seek physics-informed reduced-order nonlinear models of LiBs from measured data. The model was trained using a high-fidelity model of a Li-ion cell. We used Sequentially Thresholded Ridge regression (STRidge) optimization to determine the optimal reduced-order model. Using a validation set, we proposed an algorithm to tune hyperparameters (threshold and regularization parameters). A stochastic electrical current signal up to 2C/4C-rates charge/discharge was used in the training set, and the US highway profile (US06 drive cycle) was used for the validation. The model was validated with EPA Urban Dynamometer Driving Schedule (UDDS) as the test set. The test errors (normalized root mean square error (NRMSE)) were 6.3e-3 for SOC and Voltage predictions.
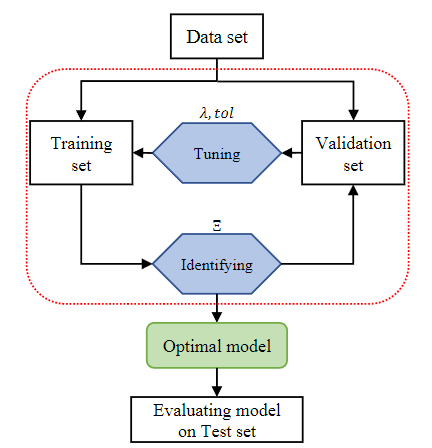
O. Ahmadzadeh, R. Rodriguez, Y. Wang and D. Soudbakhsh, “A Physics-Inspired Machine Learning Nonlinear Model of Li-ion Batteries,” 2023 American Control Conference (ACC), San Diego, CA, USA, 2023, pp. 3087-3092,
doi: 10.23919/ACC55779.2023.10156368