PROFESSIONAL SUMMARY
❖ Ph.D. candidate in Mechanical Engineering working on Modeling and Control of Li-ion batteries for Electric Vehicles (EVs) and Fast-Charging
• 4 years of academic research in modeling Li-ion batteries for accurate state-of-charge and voltage prediction (papers 5)
• 4 industry internships modeling and controlling racing yachts, complex engines, and energy storage systems (papers 3)
• Experience with Machine Learning / Deep Learning for signal and image processing in biomedical applications (paper 1)
• Modeling and control of unmanned aerial and underwater vehicles (UAV, UUV) with experience in data acquisition and data processing (paper 1)
Resume: bit.ly/Resume_Renato_Rodriguez
PERSONAL SUMMARY
Renato is currently pursuing his Ph.D. in the field of Mechanical Engineering at Temple University, Philadelphia PA. He completed his B.Sc. and M.Sc. in Mechanical Engineering at George Mason University and Temple University respectively. Renato’s research interests include control of linear and nonlinear dynamical systems, model-based and model-free optimization, data-driven modeling, and model-reduction of complex high-dimensional systems with unknown dynamics. He completed four separate internships at Ford Motor Company’s Research and Advanced Engineering (R&AE) division, where he led the research efforts on an ongoing project involving optimal control of a complex nonlinear MIMO system (AC75 sailboat) via model-free optimization and implemented data-driven modeling (DDM) techniques to develop proxy models of the complex internal combustion engine (ICE) and lithium-ion battery (LIB) systems. He is a Research and Teaching Assistant for the Dynamical Systems Laboratory (DSLab), at Temple University. Renato’s Ph.D. aims to introduce more powerful data-driven modeling techniques that can outperform and exceed the limitations of current methods.
Publications (ResearchGate, Google Scholar)
- Rodriguez, R., Ahmadzadeh, O., Wang, Y., & Soudbakhsh, D. (2023). Data-driven Discovery of Lithium-Ion Battery State of Charge Dynamics. Journal of Dynamic Systems, Measurement, and Control, 1-13, https://doi.org/10.1115/1.4064026.
- Rodriguez, R., Ahmadzadeh, O., Wang, Y., & Soudbakhsh, D. (2023, May). Discovering governing equations of li-ion batteries pertaining state of charge using input-output data. In 2023 American Control Conference (ACC) (pp. 3081-3086). IEEE, https://doi.org/10.23919/ACC55779.2023.10156114.
- Ahmadzadeh, O., Rodriguez, R., Wang, Y., & Soudbakhsh, D. (2023, May). A physics-inspired machine learning nonlinear model of li-ion batteries. In 2023 American Control Conference (ACC) (pp. 3087-3092). IEEE, https://doi.org/10.23919/ACC55779.2023.10156368.
- Rodriguez, R., Wang, Y., Ozanne, J., Morrow, J., Sumer, D., & Filev, D. (2022). Adaptive Learning and Optimization of High-speed Sailing Maneuvers for America’s Cup. Journal of Dynamic Systems, Measurement, and Control, 1-12, https://doi.org/10.1115/1.4056107.
- Rodriguez, R., Wang, Y., Ozanne, J., Sumer, D., Filev, D., & Soudbakhsh, D. (2022). Adaptive Takeoff Maneuver Optimization of a Sailing Boat for America’s Cup. Journal of Sailing Technology, 7(01), 88-103, https://doi.org/10.5957/jst/2022.7.4.88.
- Rodriguez, R., Wang, Y., Ozanne, J., Sumer, D., Filev, D., & Soudbakhsh, D. (2022). Adaptive Learning for Maximum Takeoff Efficiency of High-Speed Sailboats. IFAC-PapersOnLine, 55(12), 402-407, https://doi.org/10.1016/j.ifacol.2022.07.345.
- Ahmadzadeh, O., Rodriguez, R., and Soudbakhsh, D. “Modelling of Li-ion Batteries for Real-Time Analysis: A Data-Driven Approach”, American Control Conference, 2022, https://doi.org/10.23919/ACC53348.2022.9867616.
- Rodriguez, R. (2021). OPTIMAL CONTROL OF THE AC75 SAILBOAT FOR THE AMERICA’S CUP RACE (Master’s Thesis, Temple University. Libraries), http://dx.doi.org/10.34944/dspace/7177.
- Shawki, N., Rodriguez R., Obeid, I., & Picone, J. (2021, December). On Automating Hyperparameter Optimization for Deep Learning Applications. In 2021 IEEE Signal Processing in Medicine and Biology Symposium (SPMB) (pp. 1-7). IEEE, https://doi.org/10.1109/SPMB52430.2021.9672266.
- Rodriguez, R., & Soudbakhsh, D. (2019, October). Modeling and predictive control of an unmanned underwater vehicle. In Dynamic Systems and Control Conference (Vol. 59162, p. V003T21A009). American Society of Mechanical Engineers, https://doi.org/10.1115/DSCC2019-9154.
Presentations
- ACC 2023, “Discovering governing equations of li-ion batteries pertaining state of charge using input-output data”
- IMECE 2023, “Data-Driven Modeling for Accurate State-of-Charge Prediction of Li-Ion Batteries”
- ALCOS 2022, “Adaptive Learning for Maximum Takeoff Efficiency of High-Speed Sailboats”
- Ford Motor Co.’s 3rd Artificial Intelligence & Machine Learning Conference 2022, “Data-driven Modeling of Li-Ion Batteries”
- ECC 2022, “Adaptive Learning Optimization of A High-Speed Sailboat for the America’s Cup”
- Ford Motor Co.’s 6th Global Control Conference 2021, “Optimal Control of the AC75 Sailboat for the America’s Cup Race”
- DSCC 2019, “Modeling and predictive control of an unmanned underwater vehicle”
Research
Data-driven Discovery of Governing Equations of Li-ion Batteries Pertaining State of Charge
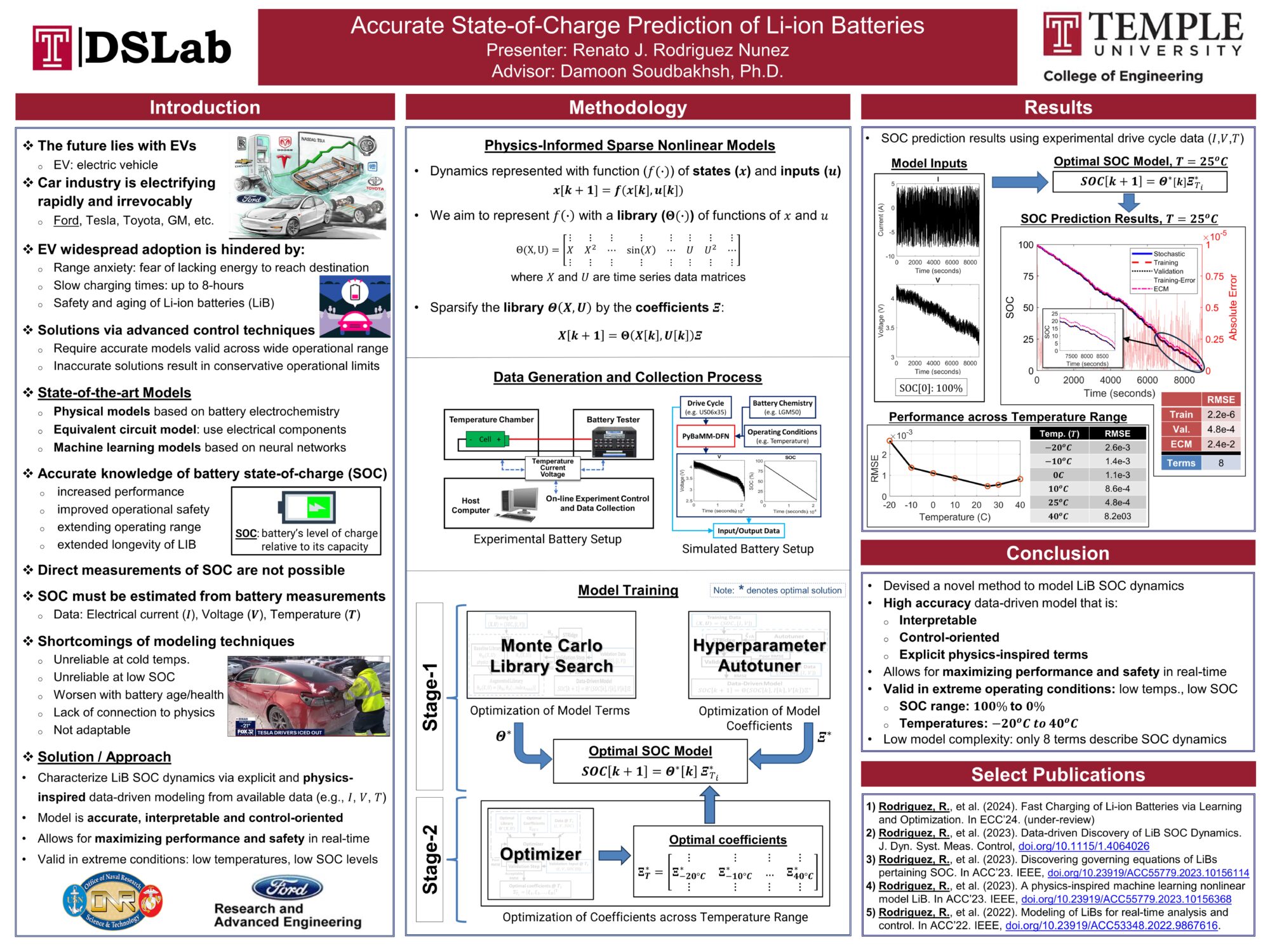
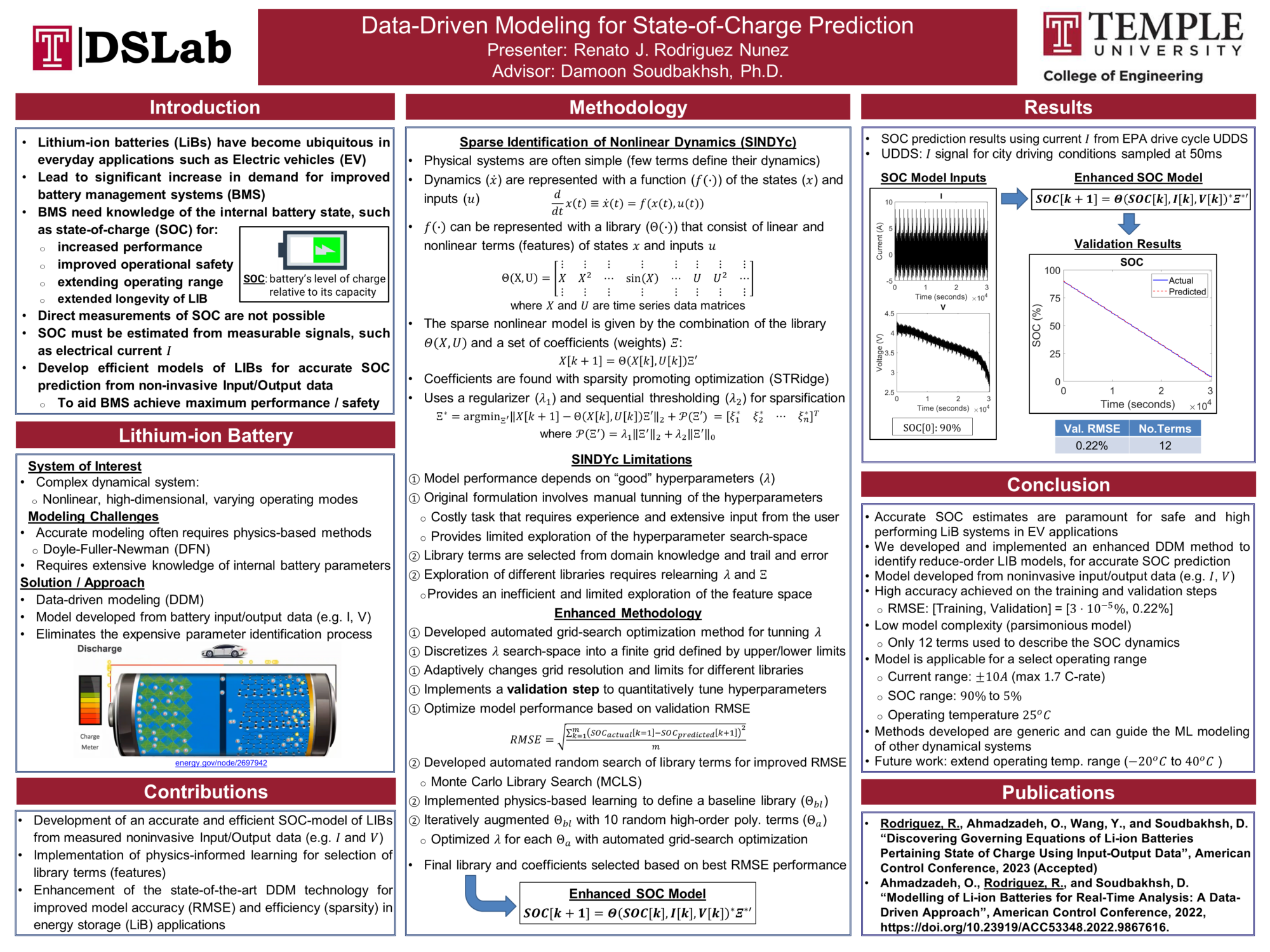
Optimal Control of an AC75 Sailboat for the America’s Cup Race
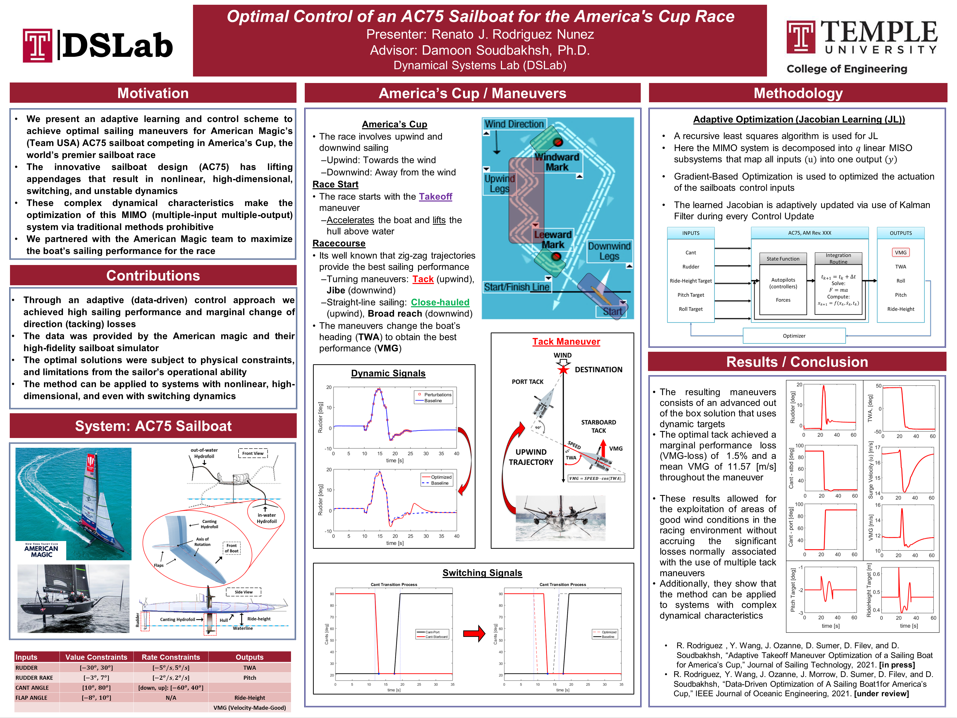
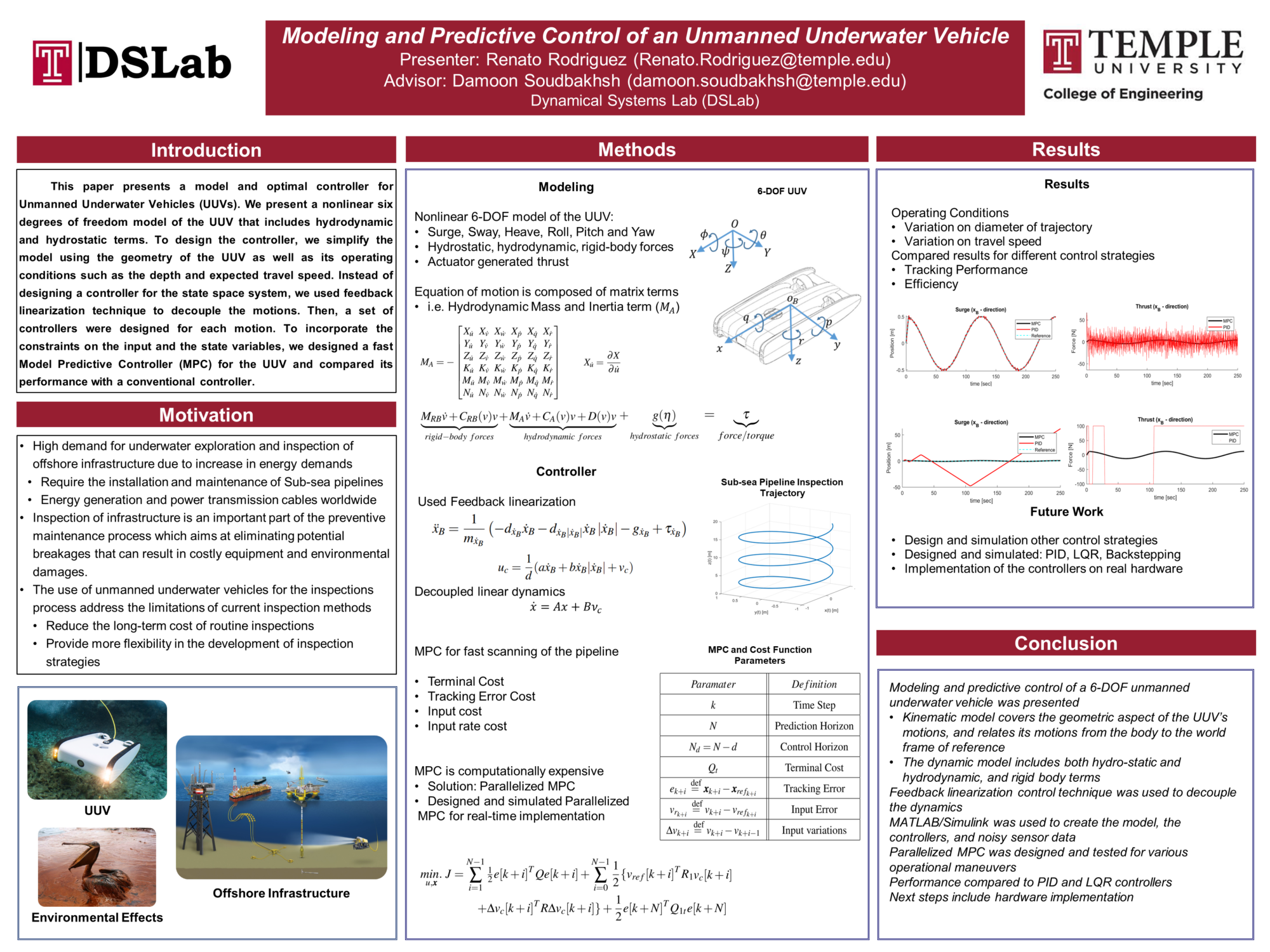